In the domain of artificial intelligence, ESG represents Environmental, Social, and Governance principles. These principles guarantee that AI development is ethical, sustainable, and transparent. On the environmental front, they focus on reducing energy consumption and minimizing e-waste. Socially, they promote diversity and inclusion to create fair AI systems. Governance ensures transparent decision-making, accountability, and compliance with regulations. By adhering to ESG principles, AI companies enhance their reputation and build trust with stakeholders. Dive deeper to see real-world examples and understand how these principles benefit AI initiatives further.
Table of Contents
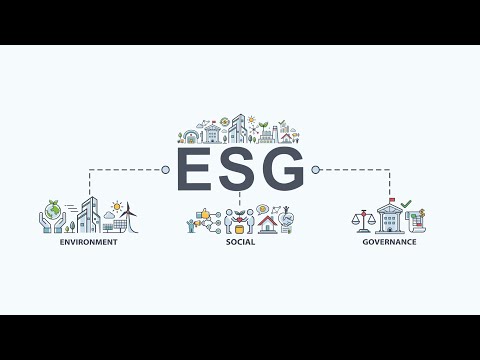
Related Video: "Environmental, Social and Governance (ESG) | Overview and Framework" by Corporate Finance Institute
Main Points
– Environmental factors: Focus on reducing AI’s carbon footprint and promoting energy-efficient practices.
– Social factors: Emphasize diversity, inclusion, and fairness in AI systems to prevent biases.
– Governance factors: Ensure transparent, accountable, and ethically compliant AI processes.
– Stakeholder engagement: Involve stakeholders in AI development to address concerns and ensure ethical use.
– Regulatory compliance: Adhere to data privacy laws and standards to build trust and avoid legal issues.
Understanding ESG Principles

Understanding ESG principles requires a clear grasp of how environmental, social, and governance factors impact ethical business practices. You might wonder how these principles come into play, especially in the domain of artificial intelligence (AI). Well, focusing on ESG means considering the broader impact your business decisions have on the world.
First, stakeholder engagement is essential. It’s not just about your shareholders but also about employees, customers, suppliers, and the community. You need to actively seek their input and address their concerns. For instance, when developing AI technologies, you should engage with various stakeholders to understand their needs and fears, ensuring that the AI solutions you create are beneficial and ethical.
Next, diversity inclusion is a cornerstone of social responsibility. Building a diverse team isn’t just about meeting quotas; it’s about bringing in different perspectives that can lead to more innovative and fair AI systems. If your team is diverse, you’re more likely to identify potential biases in AI algorithms and address them effectively.
Environmental Impact of AI

When you consider AI’s environmental impact, energy consumption is a key concern, given the extensive resources required for data centers.
Tackling e-waste management strategies is essential to minimize the environmental harm from outdated hardware.
Additionally, reducing the carbon footprint of AI operations can greatly contribute to sustainability goals.
Energy Consumption Concerns
AI systems demand substantial energy, raising notable environmental concerns. As you deploy and scale AI applications, it’s important to address their energy consumption. One effective strategy is integrating renewable sources into your energy mix. By utilizing solar, wind, or hydroelectric power, you can greatly reduce your carbon footprint. Additionally, conducting regular energy audits can help you identify inefficiencies and optimize your energy usage.
Consider the following strategies and their impacts:
Strategy | Environmental Impact |
---|---|
Using renewable sources | Lowers carbon emissions |
Conducting energy audits | Identifies inefficiencies |
Optimizing algorithms | Reduces computational power needed |
Optimizing algorithms is another practical approach. Efficient code requires less computational power, thereby consuming less energy. You shouldn’t overlook the importance of sustainable practices in AI development. By focusing on these strategies, you can make a positive environmental impact.
Balancing AI innovation with environmental sustainability isn’t just a corporate responsibility; it’s a necessity. If you’re mindful of your energy consumption, you’ll not only contribute to a healthier planet but also position your organization as a leader in sustainable technology. Don’t wait—start implementing these strategies today to make sure that your AI systems are both powerful and environmentally friendly.
E-Waste Management Strategies
Effectively managing e-waste is essential to minimizing the environmental impact of AI technologies. When you’re working with AI hardware, like servers and specialized chips, they eventually reach the end of their lifecycle. Instead of letting them pile up in landfills, you should look into robust recycling programs. These programs can reclaim valuable materials and reduce the strain on natural resources.
You also need to be mindful of proper disposal policies. Simply tossing e-waste into the trash isn’t just irresponsible; it’s often illegal. Most electronics contain hazardous substances that can leak into soil and groundwater. By following strict disposal policies, you can make certain that harmful components are handled safely and sustainably.
Furthermore, you should consider partnering with certified e-waste recyclers. These organizations specialize in safely dismantling and processing electronic waste, ensuring that nothing harmful ends up in the environment. They often provide documentation for compliance audits, helping you meet regulatory requirements.
Lastly, educating your team about e-waste management can make a significant difference. Encourage responsible practices, like reusing equipment when possible and properly disposing of outdated hardware. By integrating these strategies, you’ll help mitigate the environmental impact of your AI technologies.
Carbon Footprint Reduction
Minimizing the carbon footprint of AI technologies is essential to mitigating their environmental impact. You can make a substantial difference by adopting green computing practices and embracing carbon offsetting strategies. These efforts not only help the environment but also improve the sustainability of your AI operations.
To begin with, consider these practical steps:
– Efficient Hardware Usage: Opt for energy-efficient servers and data centers. Using hardware that consumes less power can greatly reduce the carbon emissions of your AI systems.
– Optimized Algorithms: Develop and deploy algorithms that require less computational power. Streamlining code and processes can lower the energy demands of running AI models.
– Renewable Energy Sources: Power your data centers with renewable energy. Shifting to solar, wind, or other green energy sources can significantly cut down the carbon footprint associated with AI technologies.
Don’t forget about carbon offsetting. By investing in projects that reduce or capture carbon emissions, you can balance out the carbon footprint your AI technologies produce. This proactive approach not only helps the environment but also enhances your organization’s ESG (Environmental, Social, Governance) profile.
Start integrating these practices today to lead the way in sustainable AI development.
Social Considerations in AI

When developing AI systems, you must take into account their potential social impacts to guarantee ethical and equitable outcomes. One vital aspect is data privacy. Ensuring that the data used respects individuals’ privacy is essential. You should implement robust data anonymization techniques and secure storage methods to protect sensitive information. Breaches in data privacy can lead to a loss of trust and legal repercussions.
Equally important are diversity initiatives. AI systems often reflect the biases in their training data. By promoting diversity in your data sets and development teams, you can mitigate these biases. This means not only including diverse demographic data but also fostering an inclusive environment where diverse perspectives are valued. Diverse teams are more likely to identify and address potential biases and ethical issues, leading to fairer AI outcomes.
Additionally, you need to take into account the broader societal implications of AI deployment. How will it affect employment, social interactions, and access to resources? Ensuring that AI benefits a wide range of societal groups rather than exacerbating existing inequalities is crucial. By proactively addressing these social considerations, you can develop AI systems that are both innovative and socially responsible.
Governance in AI Practices

When considering governance in AI practices, you need to focus on ethical AI decision-making and adherence to regulatory compliance standards.
It’s essential for companies to guarantee their AI systems make unbiased and fair decisions.
Additionally, staying updated with evolving regulations will help you maintain trust and avoid legal pitfalls.
Ethical AI Decision-Making
Ethical AI decision-making demands robust governance practices to guarantee responsible and fair outcomes. When you’re developing AI systems, you need to prioritize algorithmic fairness and bias mitigation to make certain that your models don’t perpetuate existing inequalities. Implementing strong governance means creating a framework that oversees every step of the AI lifecycle, from data collection to deployment.
To paint a clearer picture, consider these critical elements:
– Transparency: Make sure your AI processes are transparent. Stakeholders should understand how decisions are made and on what basis.
– Accountability: Establish clear accountability. Designate roles and responsibilities for those involved in the AI development process.
– Validation: Regularly validate your AI models. Use diverse datasets and regularly check for biases to guarantee fair outcomes.
Regulatory Compliance Standards
Following regulatory compliance standards guarantees that your AI systems not only operate ethically but also meet legal requirements, safeguarding against potential risks.
When it comes to data privacy, adhering to regulations like GDPR or CCPA is essential. You’ve got to make sure that your AI processes data in a way that respects user privacy and confidentiality. Non-compliance can lead to hefty fines and damage to your reputation.
Incorporating risk management into your AI practices is another critical aspect of governance. You should regularly assess and mitigate risks associated with your AI systems, such as algorithmic biases or cybersecurity threats. By doing so, you can avoid scenarios that might lead to legal issues or ethical dilemmas.
Don’t overlook the importance of transparent documentation. Keeping detailed records of your AI’s decision-making processes and data handling practices can be invaluable. If regulatory bodies question your compliance, you’ll have the necessary documentation to back up your claims.
Ultimately, staying on top of regulatory compliance standards not only shields you from legal repercussions but also builds trust with your users. They’ll know that your AI systems prioritize their privacy and operate within ethical and legal frameworks, making them more likely to engage with your solutions.
ESG Benefits for AI Companies

AI companies that embrace ESG principles can gain a competitive edge in today’s market. By integrating Environmental, Social, and Governance (ESG) criteria into your business strategy, you can enhance investor relations and elevate your corporate reputation. Investors are increasingly looking for companies that demonstrate a commitment to sustainability and ethical practices. By prioritizing ESG, you signal to the market that you’re forward-thinking and responsible.
Implementing ESG practices can also lead to operational efficiencies and cost savings. For example, adopting energy-efficient technologies can lower your utility bills, while promoting a diverse and inclusive workplace can improve employee satisfaction and retention. In addition, strong governance can mitigate risks and ensure regulatory compliance, making your company more resilient.
Here are some tangible benefits:
– Attracting top talent: Employees are more likely to join and stay with companies that align with their values.
– Customer loyalty: Consumers prefer brands that are socially and environmentally responsible.
– Risk management: ESG practices help identify and manage risks, reducing the likelihood of scandals or legal issues.
Challenges in Implementing ESG
Despite the clear benefits, incorporating ESG principles in your AI company can pose several challenges. One major hurdle is stakeholder engagement. You need to guarantee that all parties, from employees to investors, understand and support ESG goals. This necessitates ongoing dialogue and education, which can be time-consuming and resource-intensive.
Another significant challenge is data transparency. To truly adhere to ESG principles, you must gather, analyze, and share data on various metrics like environmental impact, social responsibility, and governance practices. This can be intimidating, especially when dealing with complex AI algorithms and proprietary technologies. Ensuring that this data isn’t only accurate but also accessible to all stakeholders adds another layer of complexity.
Additionally, aligning ESG goals with business objectives can be tricky. You might find it challenging to balance profitability with ethical considerations, especially when short-term gains conflict with long-term sustainability. This balancing act often requires strategic adjustments and sometimes even reevaluating your core business model.
Lastly, regulatory compliance is a moving target. Different regions have varying ESG standards, making it difficult to maintain consistency across global operations. Maneuvering through these regulatory landscapes demands both vigilance and adaptability, further complicating your ESG implementation efforts.
Case Studies of ESG in AI
Let’s explore real-world examples of how companies are successfully integrating ESG principles in their AI initiatives. By doing this, you’ll see the tangible impact of ESG in action.
One notable example is IBM’s diversity initiatives. IBM has been actively working to guarantee their AI systems are free from bias. They’ve introduced rigorous testing protocols to identify and eliminate biases, enhancing the fairness of their AI systems. This commitment demonstrates how diversity initiatives can directly impact AI development.
Google is another leader in this space. They’ve launched community outreach programs to educate underserved communities about AI. By offering free courses and resources, Google aims to democratize AI knowledge, ensuring broader participation and representation in the AI field.
Finally, Microsoft has implemented sustainable AI practices. They’ve committed to using AI to tackle environmental issues, such as optimizing energy use in data centers. This not only reduces their carbon footprint but also sets a precedent for using AI in environmentally responsible ways.
– IBM: Diversity initiatives to eliminate AI bias.
– Google: Community outreach programs to educate underserved communities.
– Microsoft: Sustainable AI practices to reduce environmental impact.
These case studies highlight how integrating ESG principles into AI initiatives can lead to tangible benefits.
ESG Metrics in AI Evaluation
Building on these case studies, it’s important to understand how companies measure the success of their ESG initiatives in AI. You’ll find that AI transparency is an essential metric. Companies need to make sure their AI systems are understandable and explainable. This means they should openly share how their algorithms work, what data they use, and the decision-making process behind their AI models. Transparency builds trust and helps stakeholders assess the ethical implications of AI applications.
Another key metric is community engagement. Organizations should actively involve the communities affected by their AI systems. This could include hosting public forums, seeking feedback, or collaborating with local groups to understand their needs and concerns. Such engagement ensures that AI solutions aren’t only technically sound but also socially responsible and equitable.
Environmental impact is another significant metric. Companies should evaluate the energy consumption and carbon footprint of their AI operations. This involves optimizing algorithms for efficiency and exploring sustainable energy sources.
Future of ESG in AI
The future of ESG in AI promises to integrate ethical considerations even more deeply into the development and deployment of AI technologies. As you navigate this evolving landscape, you’ll notice that companies are increasingly focused on making AI systems more transparent and accountable. This is vital for building public trust and guaranteeing that AI serves the greater good.
To paint a clearer picture, consider these key developments:
– Enhanced AI Transparency: Companies will provide clearer insights into how AI algorithms make decisions, making it easier to identify biases and correct them.
– Public Trust Initiatives: Efforts will ramp up to make sure that AI technologies are trustworthy and reliable, including third-party audits and certifications.
- Sustainable AI Practices: Organizations will emphasize creating AI solutions that are energy-efficient and environmentally friendly.
Frequently Asked Questions
How Can Small AI Startups Integrate ESG Principles Effectively?
To integrate ESG principles effectively, you should focus on employee engagement and smart resource allocation. Encourage your team to participate in sustainable practices and make sure everyone understands the importance of ESG.Allocate resources to projects that align with environmental, social, and governance criteria. By fostering a culture of responsibility and making deliberate choices, your startup can successfully incorporate ESG principles into its operations.
What Role Do Investors Play in Promoting ESG in Ai?
Did you know that 73% of investors consider ESG factors when making decisions?Your role as an investor is vital in promoting ESG in AI. By prioritizing ethical funding, you can steer AI startups towards responsible practices.Your investor influence guarantees that companies align with environmental, social, and governance standards, fostering sustainable innovation.
Can ESG Compliance Enhance AI Algorithm Transparency?
Yes, ESG compliance can enhance AI algorithm transparency. When you focus on data ethics, you're ensuring the AI operates fairly and ethically. This transparency helps build trust.Additionally, considering the environmental impact means you're optimizing AI for sustainability, which adds another layer of accountability. By adhering to ESG principles, you're making the entire AI process more transparent and responsible.