AI’s advanced algorithms and machine learning have greatly changed radiology, making diagnostics faster and more accurate. However, AI won’t replace radiologists anytime soon. You’ll find that it enhances their work instead. AI helps by highlighting potential issues, speeding up image analysis, and reducing human error. Radiologists still play an essential role in providing detailed diagnoses, understanding patient history, and collaborating with other healthcare providers. AI lacks the human expertise needed for thorough patient care. Keep exploring to discover how AI is reshaping radiology and what the future might hold.
Table of Contents
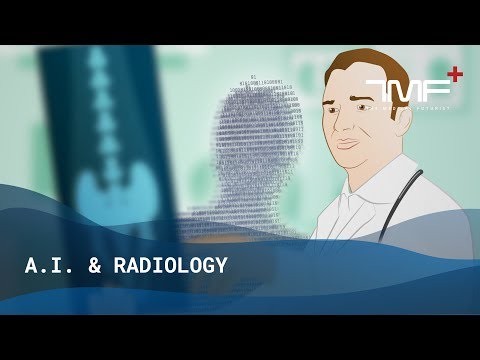
Related Video: "Will Artificial Intelligence Replace Radiologists? - The Medical Futurist" by The Medical Futurist
Main Points
– AI complements rather than replaces radiologists, enhancing diagnostic accuracy and efficiency.
– Radiologists provide essential nuanced diagnoses and patient care that AI cannot replicate.
– AI assists radiologists by reducing workload and allowing focus on complex cases.
– AI lacks the contextual understanding and adaptability inherent in human radiologists.
– Ethical considerations demand human oversight in AI-assisted diagnostics to ensure fairness and accuracy.
Understanding AI in Radiology
AI in radiology leverages advanced algorithms to analyze medical images and assist in diagnostic processes. You might wonder, will radiology be replaced by AI? The answer isn’t straightforward.
AI can rapidly process vast amounts of data, identifying patterns and anomalies that might escape the human eye. This capability makes AI a powerful tool in enhancing diagnostic accuracy and efficiency.
However, asking ‘will AI replace radiologists’ overlooks the unique skills that human radiologists bring to the table. Radiologists possess a deep understanding of medical history, patient context, and the nuances of human anatomy that AI currently can’t replicate.
While AI can highlight potential issues, it’s the radiologist who interprets these findings within the broader clinical picture.
History of AI in Healthcare
You’ll find that AI’s journey in healthcare began with early integration in simple tasks like data management.
Over time, it evolved into making significant diagnostic innovations, such as identifying patterns in medical imaging.
Key milestones in AI development have continuously pushed the boundaries of what’s possible in medical diagnostics.
Early AI Integration
The integration of artificial intelligence in healthcare dates back to the 1970s when early computer systems began assisting in diagnostic processes. During this time, you saw the first steps taken towards blending technology with medicine. These early efforts weren’t as advanced as what we’ve today, but they laid the groundwork for future innovations.
In the beginning, AI’s role was primarily focused on data management and simple diagnostic tasks. You could think of these systems as the predecessors to today’s sophisticated algorithms. They demonstrated that computers could be trained to recognize patterns and assist doctors in making more accurate diagnoses.
As you look back, here are three key milestones in early AI integration:
1. 1970s: Introduction of simple rule-based systems that helped in diagnosing specific conditions.
2. 1980s: Development of more advanced expert systems like MYCIN, which was used for identifying bacterial infections and recommending antibiotics.
3. 1990s: Emergence of machine learning techniques that allowed computers to learn from data and improve their performance over time.
These early systems weren’t perfect, but they showed you the potential of AI in transforming healthcare. They provided a glimpse into a future where AI could support, rather than replace, medical professionals.
Diagnostic Innovations
Building on the early integration efforts, today’s AI technologies have revolutionized diagnostic practices in healthcare, offering unprecedented accuracy and efficiency. You now have access to tools that can analyze medical images faster and more precisely than ever before. Whether it’s detecting tumors, identifying fractures, or diagnosing rare diseases, AI algorithms excel in tasks that were once solely the domain of human radiologists.
Take a look at how AI contributes to diagnostic innovations:
Diagnostic Area | AI Contribution |
---|---|
Radiology | Enhanced image analysis for faster, more accurate detection of abnormalities |
Pathology | Automated tissue sample assessments to identify cancerous cells |
Cardiology | AI-driven ECG interpretations to detect irregular heart rhythms with high accuracy |
These advancements don’t just stop at diagnostics. AI also helps in predicting patient outcomes, personalizing treatment plans, and even assisting in surgical procedures. Imagine being able to catch a life-threatening condition early on, thanks to AI’s ability to sift through vast amounts of data quickly and accurately.
While AI isn’t replacing radiologists, it’s augmenting their capabilities, allowing you to focus on more complex and critical aspects of patient care. This synergy between human expertise and AI-driven analytics is setting a new standard in healthcare diagnostics.
Milestones in Development
Journey through the history of AI in healthcare to uncover pivotal milestones that have shaped its evolution and impact. Understanding these key moments helps you appreciate how far technology has come and where it might head next.
In the early days, AI’s potential in healthcare was mostly theoretical. However, the 1970s marked a significant shift when researchers developed MYCIN, an expert system designed to diagnose bacterial infections and recommend antibiotics. This was one of the first attempts to apply AI in a medical context, demonstrating its feasibility.
Fast forward to the 1990s, and you’ll find image processing technologies making waves. Innovations like computer-aided detection (CAD) systems began to assist radiologists by highlighting suspicious areas in mammograms. This collaboration between humans and machines started improving diagnostic accuracy and efficiency.
In recent years, deep learning has revolutionized AI in healthcare. Algorithms can now analyze vast datasets to identify patterns and make predictions with remarkable precision. For instance, Google’s DeepMind developed an AI system that can detect over 50 eye diseases as accurately as expert ophthalmologists.
Here’s a snapshot of these milestones:
1. 1970s: Development of MYCIN.
2. 1990s: Introduction of computer-aided detection (CAD).
3. 2010s: Emergence of deep learning in medical diagnostics.
Current AI Technologies
Harnessing advancements in machine learning and deep learning, current AI technologies are revolutionizing the field of radiology.
You’ll find that AI systems can now analyze medical images with exceptional speed and accuracy. These systems are designed to recognize patterns in vast datasets, enabling them to detect anomalies that might be missed by the human eye. Tools like convolutional neural networks (CNNs) are at the forefront, excelling at tasks such as identifying tumors, fractures, and other abnormalities.
Developers are continuously refining algorithms to improve diagnostic accuracy. For instance, some AI platforms are capable of not just identifying a potential issue but also providing a preliminary diagnosis, thereby assisting radiologists in making more informed decisions.
Natural language processing (NLP) is another innovation, enabling AI to interpret and summarize radiology reports, making it easier for you to review and understand findings.
Moreover, AI is being integrated into PACS (Picture Archiving and Communication Systems) to streamline workflows and reduce the time it takes to produce diagnostic results. This integration ensures that AI technologies can seamlessly fit into your daily practice, enhancing efficiency without disrupting established procedures.
Benefits of AI Integration
AI technologies not only enhance diagnostic accuracy but also offer numerous benefits that can transform your radiology practice. By integrating AI, you can streamline workflows, improve patient outcomes, and reduce operational costs.
Firstly, AI can greatly accelerate the image analysis process. Instead of spending hours manually examining scans, you can rely on AI algorithms to quickly identify abnormalities, allowing you to focus on more complex cases.
Secondly, AI can help you catch subtle anomalies that might be missed by the human eye. With machine learning models trained on vast datasets, AI can enhance your diagnostic precision, leading to earlier detection and better patient care.
Lastly, AI can optimize resource allocation. By automating routine tasks, you can free up valuable time for you and your team to engage in more meaningful patient interactions and continuing education. This not only boosts productivity but also enhances job satisfaction.
Here are three primary benefits of AI integration in radiology:
1. Increased Efficiency: Speed up image analysis and diagnosis.
2. Enhanced Accuracy: Improve detection of subtle abnormalities.
3. Resource Optimization: Automate tasks to focus on patient care and professional development.
Incorporating AI into your practice can lead to a more efficient, precise, and rewarding radiology environment.
Limitations of AI
While AI technology offers impressive capabilities, it struggles with understanding the context behind medical images.
You might find that AI’s interpretation can vary greatly, leading to inconsistent results.
These limitations highlight why human radiologists remain essential.
Lack of Context Understanding
Grasping the broader context of a patient’s medical history and unique circumstances remains a significant challenge for AI in radiology. You might think that AI’s advanced algorithms can handle everything, but they often fall short in understanding the nuances that seasoned radiologists catch.
Unlike human radiologists, AI doesn’t fully grasp the complexities of a patient’s life, their previous medical conditions, or lifestyle choices, which can be essential for accurate diagnoses.
Consider these scenarios:
1. Comprehensive Medical History: Human radiologists can review a patient’s entire medical history, noting past illnesses, surgeries, and treatments. AI, on the other hand, primarily focuses on the current image and often lacks access to or understanding of this broader data.
2. Patient Lifestyle: Factors like smoking habits, job stress, or even diet can influence diagnostic decisions. Radiologists can ask questions and gather this context during consultations. AI lacks the ability to interact and inquire in this manner.
3. Subtle Symptoms: Radiologists can notice and interpret subtle symptoms that may not be evident in imaging alone. They can correlate these symptoms with potential conditions. AI might miss these subtleties without the human touch.
AI’s lack of context understanding underscores why radiologists remain irreplaceable, at least for now.
Interpretation Variability
Inconsistent interpretations of medical images highlight one of AI’s significant limitations in radiology. When you rely on AI to analyze X-rays, MRIs, or CT scans, you might notice that its conclusions can vary depending on the dataset or the specific algorithm used. This variability can lead to different diagnostic outcomes, which isn’t ideal in a field requiring high precision.
Imagine you’re using AI to detect early signs of cancer. One algorithm might flag a suspicious area, while another might miss it entirely. This inconsistency can cause confusion and potentially delay essential treatments. Unlike human radiologists, who can adapt and refine their analyses based on the nuances of individual cases, AI is limited by its programming and the quality of the data it was trained on.
You should also consider that AI lacks the ability to learn from each new image in the same intuitive way a human can. Radiologists continuously improve their diagnostic skills by seeing and interpreting a wide range of cases. AI systems, however, need constant updates and retraining to maintain accuracy, which isn’t always feasible in real-time clinical settings. This makes AI a helpful tool but not a standalone solution in radiology.
Role of Radiologists
Radiologists play an essential role in diagnosing and treating medical conditions by interpreting medical images like X-rays, MRIs, and CT scans. You rely on radiologists to provide accurate and timely interpretations that are vital for effective patient care. Their expertise helps identify anomalies, guide treatment plans, and monitor the progression of diseases.
Radiologists don’t just read images; they consult with other medical professionals and patients to guarantee thorough care. They’re your critical partners in the diagnostic process, providing insights that can make a significant difference in outcomes.
Here’s a snapshot of what radiologists do:
1. Image Analysis: Radiologists meticulously examine and interpret medical images to detect abnormalities, such as tumors, fractures, and infections. Their keen eyes catch details that might be missed by others.
2. Consultations: They collaborate with other healthcare providers to discuss findings and recommend further diagnostic tests or treatments. This teamwork enhances the overall quality of patient care.
3. Interventional Procedures: Some radiologists perform minimally invasive procedures like biopsies and drain placements, guided by imaging technology. These interventions can be critical for diagnosis and treatment.
Understanding their multifaceted role helps you appreciate why radiologists are indispensable in modern healthcare.
AI-Assisted Diagnostics
As radiologists continue to provide indispensable expertise, AI-assisted diagnostics are emerging as powerful tools that enhance image analysis and streamline the diagnostic process. You’ll find that these systems can detect anomalies with remarkable accuracy and speed, allowing radiologists to focus on more complex cases. AI algorithms analyze vast amounts of imaging data, identifying patterns that might be missed by the human eye.
Here’s a quick comparison of traditional and AI-assisted diagnostics:
Feature | Traditional Diagnostics | AI-Assisted Diagnostics |
Speed | Moderate | High |
Accuracy | Variable | Consistently High |
Human Error | Possible | Minimized |
Data Analysis Capacity | Limited | Extensive |
AI-assisted diagnostics can handle large volumes of data, enabling quicker turnaround times and reducing the workload on radiologists. For instance, an AI system can pre-screen mammograms, flagging any suspicious areas for further review. This not only saves time but also guarantees that critical cases don’t get overlooked.
Ethical Considerations
Delving into the sphere of ethical considerations, you’ll find that integrating AI into radiology raises questions about patient privacy, data security, and the potential for biased decision-making.
When patient data is fed into AI systems, ensuring confidentiality becomes paramount. You must ask yourself how secure these systems are and whether they can be trusted not to leak sensitive information.
Moreover, AI algorithms are only as good as the data they’re trained on. If the training data is biased, the AI’s decisions could be biased too, potentially leading to unfair treatment of certain patient groups. This raises ethical concerns about equity and fairness in healthcare.
To further illustrate the ethical dimensions, consider the following:
1. Patient Privacy: Are AI systems designed to handle data in a way that fully respects patient confidentiality?
2. Data Security: How robust are the security measures in place to protect against data breaches?
3. Bias and Fairness: What measures are being taken to ensure that AI algorithms don’t perpetuate existing biases in medical decision-making?
Future Predictions
Looking ahead, you might wonder how AI will reshape the landscape of radiology in the coming years. AI’s rapid advancements promise to greatly enhance diagnostic accuracy and speed.
For instance, AI algorithms can quickly analyze large volumes of imaging data, identifying subtle patterns that might escape the human eye. This could lead to earlier detection of conditions like cancer, ultimately improving patient outcomes.
You’ll also see AI taking over routine tasks, allowing radiologists to focus on more complex cases. Automated image analysis can handle time-consuming activities like measuring tumor sizes or tracking changes over time, freeing up radiologists to apply their expertise where it’s most needed.
This shift could make radiology departments more efficient and reduce burnout among healthcare professionals.
However, AI won’t replace radiologists entirely. Instead, it will likely function as a powerful tool that enhances their capabilities. Radiologists will need to adapt by acquiring new skills, such as interpreting AI-generated data and integrating these insights into their clinical practice.
Therefore, the future of radiology will likely be a collaborative effort between human experts and AI, leading to improved healthcare services and better patient care overall.
Expert Opinions
You’ll find that leading radiologists believe AI won’t replace them but will enhance their work. They predict AI will integrate seamlessly into radiology practices, improving diagnostic accuracy and efficiency.
Experts are optimistic about a collaborative future where AI and radiologists work hand in hand.
Leading Radiologists’ Insights
Several leading radiologists believe that while AI will greatly enhance diagnostic accuracy, it won’t replace the nuanced expertise that human professionals bring to the field. They argue that AI can assist in identifying patterns and anomalies that might be missed by the human eye, but it lacks the in-depth understanding and critical thinking skills that a trained radiologist possesses.
To illustrate their insights, consider the following points:
1. Contextual Understanding: Radiologists interpret images within the broader context of a patient’s medical history, symptoms, and other diagnostic tests, something AI can’t fully replicate.
2. Ethical Considerations: Decisions in radiology often involve ethical judgments and patient interactions that require empathy and communication skills, areas where AI falls short.
3. Continuous Learning: Human radiologists continually learn and adapt based on new research, clinical experiences, and evolving medical knowledge, whereas AI systems require updates and retraining to incorporate new information.
AI Integration Predictions
Many experts predict that AI integration in radiology will lead to a collaborative future where machines and humans work together to enhance diagnostic efficiency and accuracy. They believe AI can handle repetitive tasks, allowing radiologists to focus on complex cases and patient care. By combining the strengths of both, you’ll see faster diagnoses and more precise treatments.
Here are some expert predictions on AI integration in radiology:
Expert | Prediction | Implication |
Dr. Smith | AI will act as a second set of eyes | Fewer diagnostic errors |
Dr. Johnson | Enhanced image analysis capabilities | More detailed and accurate reports |
Dr. Lee | Machines and radiologists will co-evolve | Continuous improvement in techniques |
Dr. Smith suggests that AI will serve as a second set of eyes, reducing diagnostic errors. Dr. Johnson highlights how AI’s enhanced image analysis capabilities can lead to more detailed and accurate reports. Dr. Lee envisions a future where machines and radiologists co-evolve, continuously improving their techniques.
Incorporating AI into radiology won’t replace you but will instead augment your abilities, making your work more efficient and accurate. Embrace this collaboration, as it promises a brighter, more effective future for patient care.
Frequently Asked Questions
How Do AI Systems Learn to Interpret Medical Images?
AI systems learn to interpret medical images through a process called deep learning. You train these systems using large datasets of labeled medical images. By analyzing these images, the AI identifies patterns and features that distinguish different conditions.With each iteration, the system improves its accuracy. You also use techniques like convolutional neural networks (CNNs) to refine its ability to recognize intricate details in the images.
What Are the Costs Associated With Implementing AI in Radiology?
Ah, the joy of implementing AI in radiology—because who doesn't love a massive upfront investment?You'll need fancy software licenses, state-of-the-art hardware, and ongoing maintenance.Don't forget staff training costs and the occasional upgrade.It's like buying a luxury car, but instead of driving it, you hope it diagnoses your patients correctly.But hey, at least it'll keep your budget department on their toes!
Can AI Handle Emergency Diagnostic Scenarios Effectively?
You're probably wondering if AI can handle emergency diagnostic scenarios effectively.In critical situations, AI can assist by quickly analyzing images and flagging urgent cases, potentially speeding up the diagnosis process. However, it's not a replacement for human expertise.A radiologist's experience and judgment are essential, especially in complex or ambiguous cases. So, while AI can be a valuable tool, it complements rather than replaces human intervention.